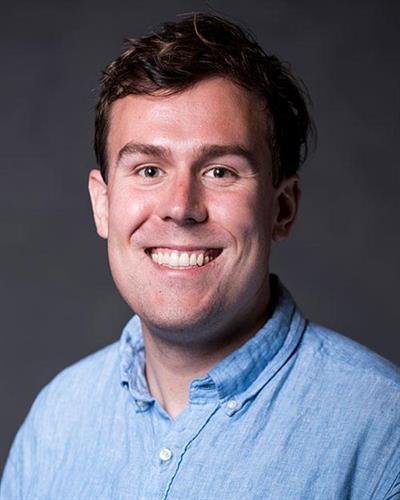
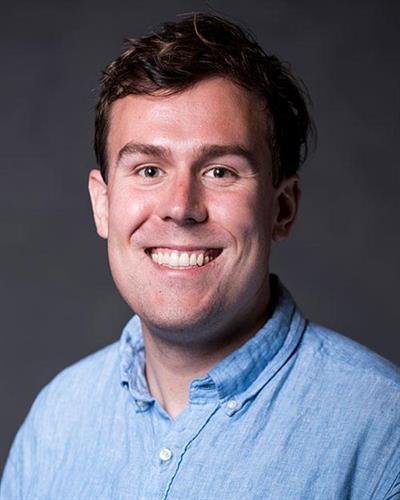
Research/Areas of Interest:
Machine learning, harmonic analysis, statistical learning, graph theory, data science, computational mathematics, image processing, signal processing
Education
- PhD, Univ Maryland, USA, 2015
- MS, Univ Maryland, USA, 2013
- BS, University Chicago, USA, 2011
Biography
My research spans pure mathematics, computational mathematics, and applications to remote sensing, image processing, and analysis of medical data.
Mathematically, my interests are in harmonic analysis, machine learning, and theoretical statistics. My Ph.D. work developed new representation systems (frames) for certain classes of functions. This line of research is in the tradition of Fourier and wavelet analysis, but with an eye towards incorporating directionality into the representation system. Recently, my theoretical work has focused on machine learning theory, specifically unsupervised learning. I am interested in spectral graph methods for unsupervised learning, including spectral clustering and the impact of incorporating non-Euclidean metrics into unsupervised learning problems. Relatedly, I study models for random and data-driven graphs and the behavior of eigenfunctions of operators on such graphs, which has analogies to classical Fourier analysis. This line of research combines graph theory, harmonic analysis, and statistics. This research is used to prove rigorous performance guarantees on machine learning methods.
I also develop efficient algorithms for data analysis. Recent projects focus on efficient, scalable methods for unsupervised learning. A general goal for this body of work is to produce algorithms that are independent or only weakly dependent on the ambient dimension of the data, and scale quasilinearly in the number of sample points. These considerations are necessary to handle real-world datasets, which can easily consist of millions or more samples in hundreds of dimensions. I am a proponent of open-source software, and I freely release toolboxes for the methods I develop.
Beyond mathematics and computation, I am interested in applications of machine learning and computational harmonic analysis to an array of scientific disciplines, including remote sensing and medicine. A rich source of data is hyperspectral imagery (HSI), which consists of a sequence of images captured at narrow electromagnetic ranges. This data source provides detailed information about the scene being imaged, but is very high-dimensional and noisy, and requires sophisticated methods to properly analyze. I have developed clustering and active learning methods for HSI, in addition to image registration, superresolution, and fusion algorithms for a range of remote sensing data modalities. I also collaborate with medical scientists to analyze electrocardiogram (ECG) signals from patients with heart disease, in order to develop new diagnostic and predictive tools. These methods are essentially unsupervised, and learn latent structure in the patients which can be used to gain a greater understanding of the variability in human cardiac states, as well as provide predictive tools for future illness.