Tufts advances offshore wind energy engineering
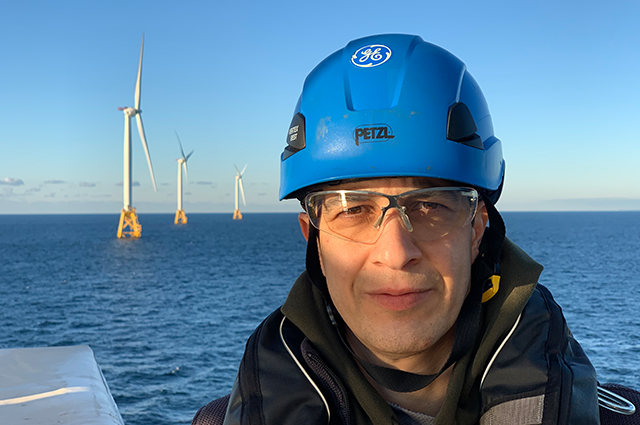
With only seven offshore wind turbines (OWTs) operating in U.S. waters compared to almost 6,000 in European waters, the U.S. is severely lagging in global offshore wind infrastructure. At this critical juncture as the negative effects of climate change and dependence on fossil fuels continue to mount, Tufts School of Engineering has become a leader in offshore wind energy engineering, with research, teaching, policy collaborations, and academic programs dedicated to innovation in the field.
With a flagship scholarly article recently published in Renewable Energy, Tufts is paving the way for the U.S. transition to offshore wind energy. A Tufts team including Professor of the Practice Eric Hines and Professor Babak Moaveni, both of the Department of Civil and Environmental Engineering, and Tufts affiliate Mingming Song, is collaborating with the University of Rhode Island, Rhode Island Coastal Resources Management Council, and the Norwegian Geotechnical Institute to develop a sustainable U.S. offshore wind infrastructure. The group's efforts are detailed in the paper, which outlines a continuous monitoring system installed in the first commercial offshore wind farm in the United States.
Faculty members across the School of Engineering are committed to advancing this important field of research. Moaveni’s recent presentation at the GE EDGE conference, titled “Digital Twinning of Block Island Offshore Wind Turbine using One Year of Monitoring Data,” was awarded as one of the best presentations at the conference in the Controls and Optimization track. Moaveni’s research has long focused on structural health monitoring, including monitoring and assessment of offshore wind energy engineering. During the height of the COVID-19 pandemic, European team members were unable to travel to the U.S. In their absence, Moaveni went through a rigorous training course required to be allowed on the offshore turbines and helped install a monitoring system comprised of vibration sensors as part of a federally funded project.
Moaveni and three co-PI’s – Professor Usman Khan of the Tufts Department of Electrical and Computer Engineering, Senior Data Scientist Anna Haensch from Tufts University Data Intensive Studies Center, and Assistant Professor Hamed Ebrahimian of the University of Nevada, Reno – are collaborating with Professor of the Practice Eric Hines and Professor Dan Kuchma of the Department of Civil and Environmental Engineering, Associate Professor Beth Rosenberg of Tufts School of Medicine, Professor of the Practice Bobbi Kates-Garnick of The Fletcher School, Professor Bruce Boghosian of the Department of Mathematics, and Associate Professor Bryony DuPont of Oregon State University. The team recently received a $1.48 million grant from the National Science Foundation (NSF) to improve offshore wind energy infrastructures in the U.S. The team hopes to optimize infrastructure, which will result in a stronger U.S. clean-energy system and a better defense against climate change worldwide. “As OWTs grow, the reliability of such massive structures is a key consideration to ensure the availability of low-cost renewable energy,” according to the team’s proposal.
Most offshore wind turbines (OWTs) are built to last 25-35 years. As the turbines age, they need to be decommissioned, rebuilt, or retrofitted. Moaveni and team propose to develop a joint modeling framework to assist with this decision-making process. Factoring in key areas such as safety, policy, and labor, the program can perform short- or long-term modeling to help understand the impact of decisions related to OWTs.
The framework will take the form of customizable computer models of OWTs or OWT systems. Those models, called digital twins, would be updated based on current data about the turbines. According to Moaveni and team, “the integration of safety and policy into offshore wind models will drive much needed change in the clean energy industry.”
Although the project will focus mainly on OWT systems in U.S. waters plus one in the United Kingdom, the improved resiliency of offshore wind infrastructures has global implications. Additionally, the team will leverage expertise from offshore wind workers, policy makers, and more from around the world to ensure that the project takes a range of perspectives into account.
In addition to the essential work of improving OWT infrastructure, the project includes crucial opportunities for education and community engagement. At the research level, the grant will provide funding for one postdoctoral and several graduate and undergraduate researchers working on the project. The work will also include K-12 educational opportunities and community outreach efforts about offshore wind energy. The team aims to not only ensure a more resilient future for OWTs through improving current and future models, but also to foster the next generation of workers, policymakers, and innovators to understand the significance of offshore wind energy infrastructure.
With master’s, PhD, and certificate programs now available in offshore wind energy engineering, Tufts continues to lead the way in this interdisciplinary field. As demonstrated by the broad range of contributors to the grant, offshore wind energy engineering at Tufts encompasses many areas of study including public health, policy, civil and environmental engineering, electrical and computer engineering, math and more. As the first offshore wind graduate programs dedicated to infrastructure, supply chain, and transmission, Tufts students gain a unique experience that positions them to become leaders in renewable energy.
Learn more about Tufts’ offerings in Offshore Wind Energy Engineering.
The content of this article is solely the responsibility of the authors and does not necessarily represent the official views of the National Science Foundation. Research reported in this article was supported by the National Science Foundation, under award number 2230630.
Department:
Civil and Environmental Engineering